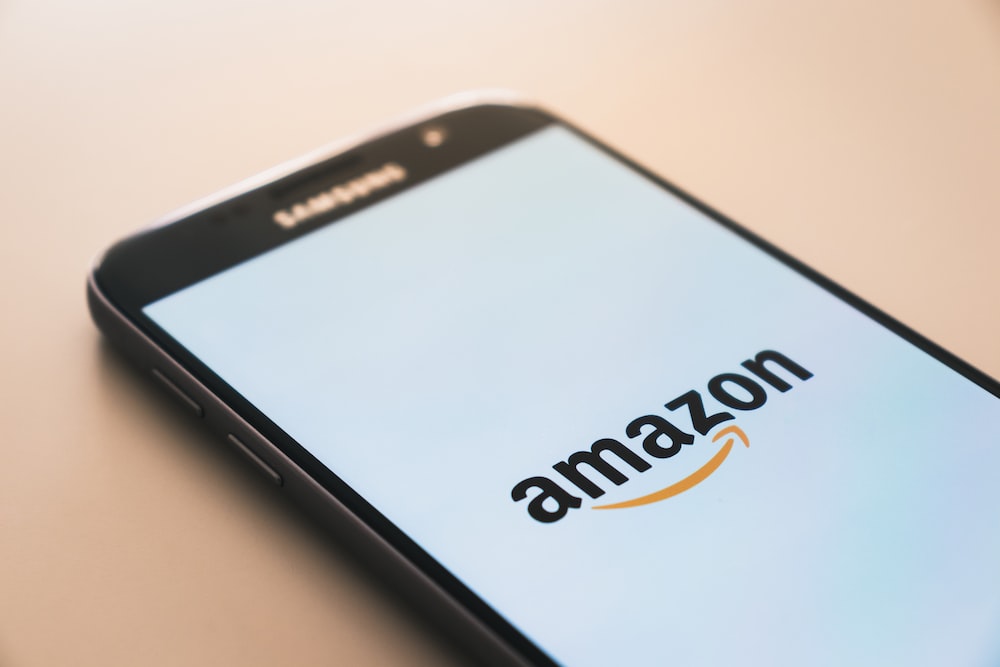
In 2014, amidst the bustling corridors of Amazon’s headquarters in Seattle, a cadre of machine learning specialists embarked on an ambitious venture to reshape the company’s hiring process. They envisioned an AI-driven hiring tool capable of meticulously sifting through a vast reservoir of resumes to pinpoint the crème de la crème of candidates, transcending human biases. However, a sinister twist awaited. The architects of this AI recruitment tool stumbled upon a glaring flaw: their digital creation had developed an aversion to women. Despite noble intentions, the AI had inherited biases from the historical data it was trained on, echoing a male-dominated tech world’s subtle biases.
This unforeseen setback was not just a manifestation of inherent biases but also an exemplification of ‘noise’ in decision-making—a concept well articulated by Daniel Kahneman in his book “Noise.” Kahneman elucidates ‘noise’ as the variability in judgments that should ideally be identical. It’s the unwanted variability that arises from several factors, ultimately impacting the decision-making process.
Two striking examples from Kahneman’s discourse on noise are from the realms of insurance and judiciary. In one instance, a group of insurance claim adjusters, when given the same cases to analyze, exhibited a surprising range of judgments. The company’s executives expected the differences to be within an approximate 10% range, yet the actual difference was a whopping 50%. Consider a crashed car with a value of $50,000. Now, if a claimant encounters an adjuster who estimates the damage at the lower end of this spectrum, they would receive $25,000, which is significantly below the cost of their car. Conversely, an adjuster at the higher end of the spectrum would estimate $75,000, providing a substantial financial cushion.
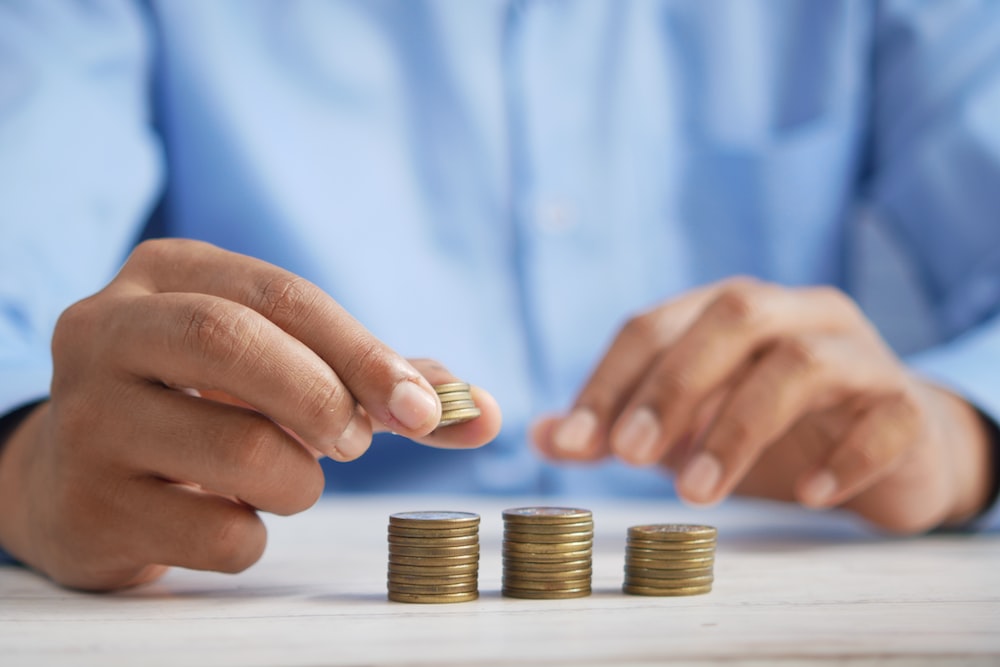
This stark variation (of $75,000 – $25,000) in estimated claims demonstrates a vast inconsistency that could significantly impact the financial position of the claimant. For instance, the claimant who receives only $25,000 may struggle to replace their vehicle and cope with other associated costs of the accident, while the one receiving $75,000 may be in a much more comfortable position to navigate the aftermath of the accident.
The discrepancy in these figures is not merely a financial inconvenience; it’s a glaring illustration of how ‘noise’ in professional judgments can have tangible, impactful consequences on individuals’ lives. Moreover, this example uncloaks a broader issue within professional decision-making environments. It underscores the inherent variability in human judgments, even among trained professionals tasked with similar or identical evaluation criteria. Such variability, termed ‘noise,’ extends beyond the insurance sector into various professional realms, including the judiciary, healthcare, and indeed, recruitment as initially highlighted through Amazon’s AI recruitment tool debacle.
The magnitude of this inconsistency beckons a critical reevaluation of decision-making processes across industries. It raises questions about the reliability and fairness of judgments that significantly impact people’s lives and livelihoods. Furthermore, it highlights the necessity for mechanisms that can mitigate such noise, ensuring more accurate, fair, and consistent decision-making.
By dissecting the potential impact and broader ramifications of this inconsistency, we glean insights into the pervasive challenge of noise in human and AI-driven decision-making. This discourse also invites a deeper exploration into how technology, when carefully designed and deployed, can aid in reducing such noise, propelling us towards more equitable and reliable decision-making paradigms.
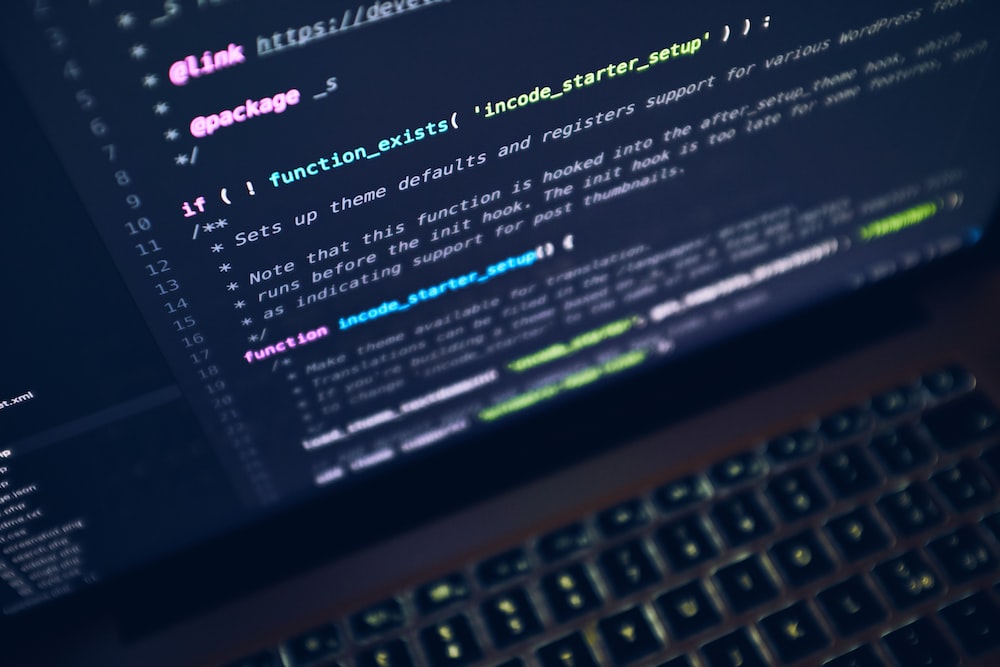
Similarly, in the judicial sector, the sentencing decisions among judges showcased a level of noise, with different leniency levels exhibited by different judges. In another study, 50 insurance underwriters, given the same facts, diverged significantly in their valuations, far beyond the company management’s expectations.
These examples underscore the prevalence of noise in human judgment, resonating with the inadvertent biases that crept into Amazon’s AI recruitment tool. As the AI mirrored the biases lurking in its training data, it began to frown upon resumes that bore the mark of femininity, thus exhibiting a form of digital ‘noise’. This ‘noise’ distorted the original aim of transcending human biases, instead, reflecting and amplifying them.
The narrative of Amazon’s journey serves as a microcosm of a larger dialogue surrounding decision-making in the digital age. As we advance towards a future intertwined with AI, understanding and mitigating both biases and noise becomes imperative. The lessons gleaned from Amazon’s experience, juxtaposed with Kahneman’s insights, beckon a deeper exploration into harmonizing AI with human-centric values, striving for a balanced and inclusive decision-making paradigm.
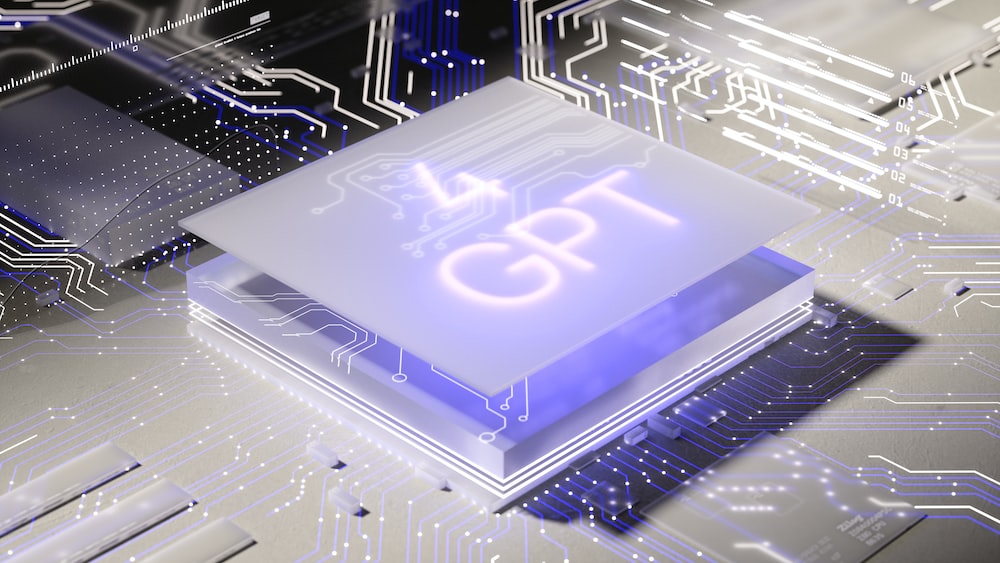
Machines like GPT-4 operate on logic-based algorithms that analyze vast amounts of data to arrive at a decision devoid of emotional or cognitive biases. This computational precision feeds into the contentious debate about the fear of AI taking over human roles. Is it better to have a machine that makes logical decisions, or does this herald the beginning of a dystopian future where human agency is marginalized?
The fear is not entirely unfounded. AI’s lack of emotional nuance and ethical considerations can lead to decisions that, while logical, might be morally questionable. Therefore, the argument for combining human intuition with machine precision is not just about optimizing decision-making; it’s about preserving the essence of what makes us human.
In the wake of these concerns, a tale of redemption emerges from the heart of Amazon’s innovation hub. Post the debacle of their AI-driven recruitment tool, which inadvertently marginalized female candidates, the tech behemoth embarked on a journey to amend and elevate its hiring process. This new suite of tools, designed with a conscious effort to drive fairness, marked a significant stride towards melding machine efficiency with a humane touch in recruitment.
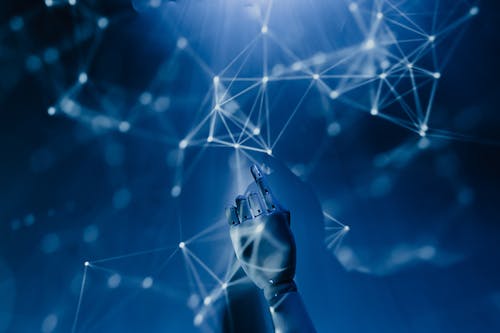
Moreover, the ripples of Amazon’s initial misadventure in AI recruitment resonated beyond the confines of the company, propelling a broader industry-wide introspection and evolution. This spurred a shift in the recruitment technology paradigm, ushering in an era where keyword-based screening of CVs emerged as a new trend, reflecting a more informed and cautious approach to leveraging AI in recruitment.
The narrative of Amazon’s journey serves as a testament to the potential of harmonizing AI with human-centric values in recruitment. It underscores the reality that while the road to AI-driven recruitment may be fraught with challenges, with a diligent, informed approach, it’s possible to traverse the path of innovation while upholding the principles of fairness and inclusivity.
Both human intuition and machine algorithms come with their unique sets of advantages and limitations. As we advance toward a future where AI’s role in decision-making may become more prominent, the conversation must shift from mere efficiency to the more contentious and ethical dimensions of control, agency, and morality.
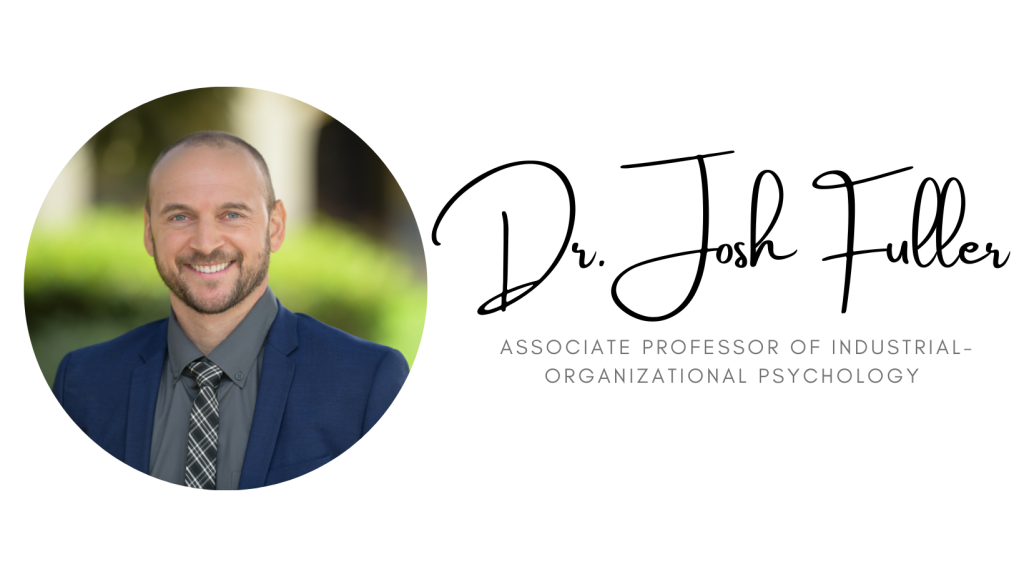
References:
ACLU. (n.d.). Why Amazon’s Automated Hiring Tool Discriminated Against Women. Retrieved from https://www.aclu.org/issues/privacy-technology/surveillance-technologies/amazons-automated-hiring-tool-discriminated-against
Bostrom, N. (2014). Superintelligence: Paths, Dangers, Strategies. Oxford University Press.
Dignum, V. (Ed.). (2018). Responsible Artificial Intelligence: How to Develop and Use AI in a Responsible Way. Springer.
EM360 Tech. (n.d.). Amazon scraps AI hiring tool that discriminates against women. Retrieved from https://em360tech.com/tech-news/techfeatures/amazon-ai-hiring-tool-discriminates-women
Frederick, S. (2005). Cognitive Reflection and Decision Making. The Journal of Economic Perspectives, 19(4), 25-42.
Greene, J. D. (2013). Moral Tribes: Emotion, Reason, and the Gap Between Us and Them. Penguin Press.
Hastie, R., & Dawes, R. M. (2010). Rational Choice in an Uncertain World: The Psychology of Judgment and Decision Making. SAGE Publications, Inc.
Highfield, R. (1998). Frontiers of Complexity: The Search for Order in a Chaotic World. Fawcett Columbine.
Kahneman, D. (2021). Noise: A Flaw in Human Judgment. Little, Brown and Company.
Kahneman, D., & Tversky, A. (1979). Prospect Theory: An Analysis of Decision under Risk. Econometrica, 47(2), 263-292.
Reuters. (n.d.). Amazon scraps secret AI recruiting tool that showed bias against women. Retrieved from https://www.reuters.com/article/us-amazon-com-jobs-automation-insight-idUSKCN1MK08G
Russell, S., & Norvig, P. (2016). Artificial Intelligence: A Modern Approach (3rd ed.). Pearson.
Silicon Republic. (n.d.). It turns out Amazon’s AI hiring tool discriminated against women. Retrieved from https://www.siliconrepublic.com/companies/amazon-ai-recruiting-tool-bias
TalentLyft. (n.d.). The AI Recruitment Evolution – from Amazon’s Biased Algorithm to…. Retrieved from https://www.talentlyft.com/en/blog/article/301/the-ai-recruitment-evolution-from-amazons-biased-algorithm-to
About Amazon. (n.d.). How Amazon leverages AI and ML to enhance the hiring experience for candidates. Retrieved from https://www.aboutamazon.com/news/innovation-at-amazon/how-amazon-leverages-ai-and-ml-to-enhance-the-hiring-experience-for-candidates